Democratization of AI needs heterogeneous compute and open ecosystem
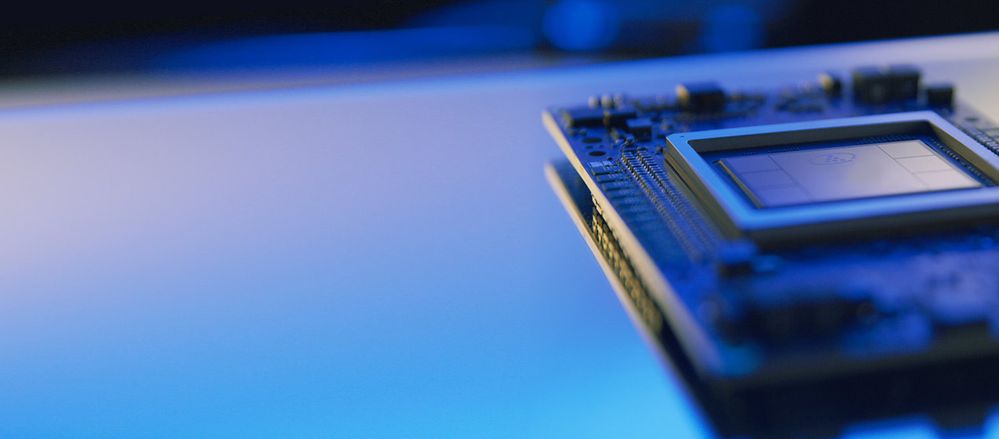
Author: Simon Chan, Managing Director of Southeast/South Asia, Australia, and
New Zealand, Sales, Marketing & Communications Group at Intel
Corporation
Earlier this year, we held Intel Vision – a series of closed-door events where we shared Intel’s next generation of technology, and how we can enable our customers and partners to succeed in the digital age. One of the hottest topics discussed at the event was artificial intelligence (AI).
Despite all the hype, AI is not new. AI has been working alongside humans for a number of years now, from reducing manufacturing errors to helping users take better pictures on a smartphone. It is well-known that AI can revolutionize industries and improve lives in countless ways.
Yet, AI has dominated global headlines in recent months, thanks to the rise of ChatGPT – a generative AI application that takes vast amounts of data it ingests to mimic human-created content. What ChatGPT has done is make it easy for people to understand the power of AI, and that it is at everyone’s fingertips to explore.
As a result, this new wave of AI has propelled many businesses to look for ways to boost their AI capabilities. Indeed, AI is no longer just about voice assistants. It has become more engaging and can help people in different ways such as code a website, or create new music and art.
According to the report 2022 Government AI Readiness Index, conducted by Oxford Insights in collaboration with the Canadian International Development Research Centre, Vietnam’s artificial intelligence readiness index has reached 53.96 out of 100, and surpasses the global average of 44.61. So, Vietnam has the potential to deploy AI to capture the opportunity to transform and develop the economy.
At the end of June 2023, in the “Artificial Intelligence: Opportunities, Challenges, and Application Potential in the Capital’s Digital Economic Development” seminar, Dr. Le Xuan Rao, Chairman of the Hanoi Union of Science and Technology Associations, said that Vietnam would focus on developing AI technology as a spearhead, which is predicted to become the most breakthrough technology sector in the next ten years.
In fact, many local businesses are using AI to enhance productivity. In the healthcare field, VinBigData has utilized AI to assist doctors in diagnosing diseases more effectively. In the banking sector, many banks have applied AI to improve information verification and customer identification through eKYC system.
However, to deploy AI successfully, we will need to solve the complexities of the compute’s requirements. From consumer electronics to the edge and cloud, compute demand will only soar as AI takes off.
Democratization of AI starts with compute
AI models in the future will only ingest ever larger amounts of data to generate useful responses for businesses. To start off on the right foot, organizations must begin with the business challenge or outcome that they are trying to solve or achieve, and leverage AI in an efficient and cost-effective way with the right compute and software required to enable it.
All these can only be meaningful if AI happens in real-time with accuracy, and compute is essential to providing the speed and performance needed to train models, make decisions or predictions, perform image and speech recognition, and scale AI systems. Think of compute as the “brains” that help machines make sense of the world and decide the actions they take next.
This is why, as AI and its algorithms advance, so must the “brains” to boost the capabilities needed. They not only need to accelerate the performance of AI, but also do so in a more efficient, secure, scalable and sustainable manner. To achieve this and democratize AI, heterogeneous compute and an open ecosystem for different AI scenarios are crucial.
AI needs heterogeneous compute for better performance, cost and energy efficiency
Ever-faster speed and performance will be the default expectation of AI from its users in the future. While this means the demand for compute power will grow exponentially, it would not make sense if businesses simply added more Central Processing Units (CPUs) and Graphics Processing units (GPUs), or build more data centers to enable AI.
To power AI’s proliferation, there are two key areas that organizations must consider. First, the identification of the type of AI workload needed. Is it for an AI chatbot to interface with customers, a large generative AI model like ChatGPT to create new content, an image recognition solution to find defects, or some other types of AI workload? And second, costs are also an important consideration to determine whether the AI solution can be easily accessed by all.
Contrary to conventional belief that all AI workloads require GPUs; the reality is that often an alternative, more efficient way to get some AI powered tasks done is with general purpose CPUs – the same ones that are already powering many of today’s data centers.
Take for example the workload of training a language model such as GPT-3. Training such large language models can cost millions of US dollars for a single model, yet most organizations will likely not need to get to that scale and instead require training much smaller models. In fact, most organizations will only need to use pre-trained models and fine-tune them with their own smaller curated data sets, and this fine-tuning can be accomplished in only minutes using Intel AI software and other standard industry open-source software, running on general purpose CPUs.
In the scenario where there indeed is a need to train a large language model, dedicated AI accelerators such as Intel’s Gaudi2 presents an alternative to traditional GPUs. In fact, Gaudi2 provides competitive cost advantages to customers, both in server and system costs. The accelerator’s MLPerf-validated performance plus upcoming software advances make Gaudi2 an extremely compelling price/performance alternative to GPUs like Nvidia’s H100.
Hence, solving the AI challenge requires a holistic approach that accounts for vastly different use cases, workloads, and power requirements. This means that different AI applications will require different compute configurations that are purpose-built with high precision, and could comprise of a diverse mix of architectures and hardware that could run the gamut of CPUs, GPUs, Field Programmable Gate Arrays (FPGAs) or other accelerators.
In short, there is no one-size-fits-all when it comes to compute, and it becomes more important than ever that the compute platform is flexible and scalable for the changing workload requirements to reach AI practicality.
AI needs an open ecosystem
Now, while we talk a lot about chips, AI is also a software problem. To democratize AI, we need an open ecosystem, and software is key to unleashing the power and scalability of AI. Without an optimized range of software frameworks and toolkits to support the hardware running AI workloads, the performance out of the box would not be able to meet optimal business requirements.
Developers need a build-once-and-deploy-everywhere approach with flexible, open and energy-efficient solutions that allow all forms of AI. One such tool is Intel’s oneAPI Toolkits, which enable businesses to write code once and run it on a variety of hardware platforms.
Tools such as this helps businesses maximize the performance of their AI workloads while minimizing the cost and complexity of managing multiple hardware platforms. AI based on an open ecosystem makes it more broadly accessible and cost-effective. It removes roadblocks that limit progress, and enables developers to build and deploy AI everywhere while prioritizing power, price and performance, using the hardware and software that best make sense for them for each job.
Investing in the future of AI
Without a doubt, AI is becoming more powerful, and has opened up new possibilities that many businesses are seeing for the first time. Whether businesses are running their AI in the cloud or creating their own on-premises solutions, they should be ready for a future where compute demand will skyrocket.
And AI will require a foundation to support different facets of AI model designs, development and deployment across different compute platforms as they continue to evolve.
To truly reap the benefits of AI, however, depends on how businesses invest in the capabilities required to get the best out of AI – and a heterogeneous compute environment and an open ecosystem that protect and future-proof current investments will be important as businesses prepare for what is next with AI.