Federated learning – the key to realizing smarter, more secure healthcare
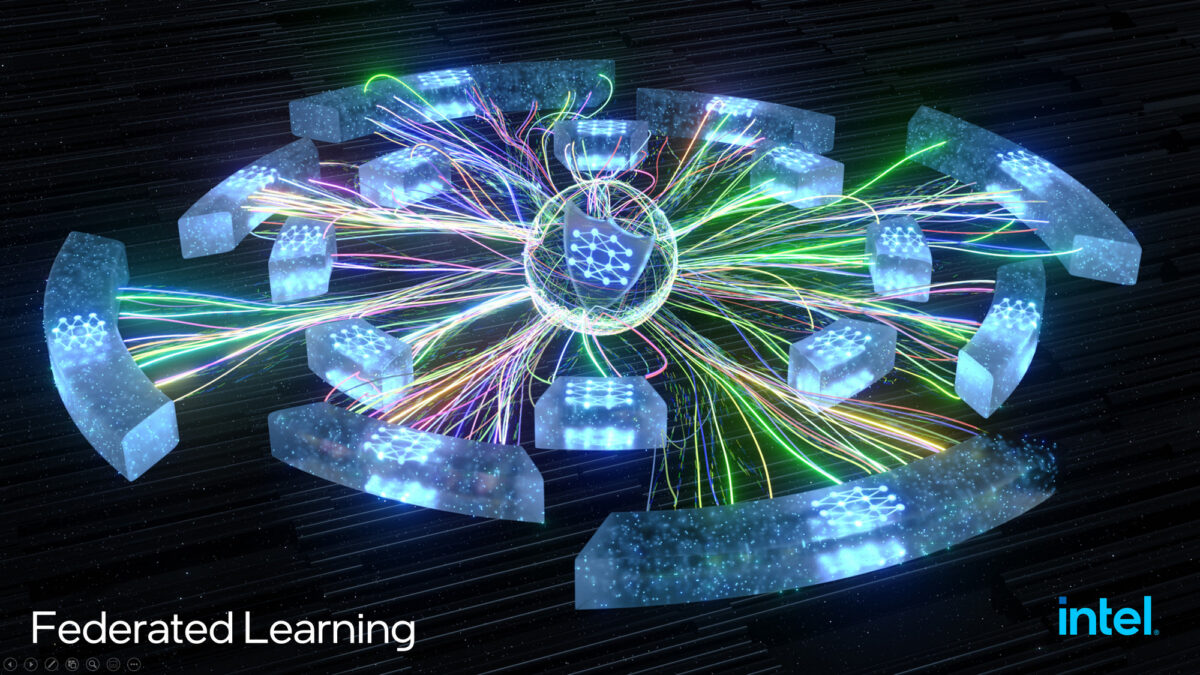
By Alexis Crowell, Vice President of Sales, Marketing & Communications Group; Managing Director – Asia Territory, Intel Corporation
Modern healthcare has become smarter, benefiting from the use of technology like Artificial Intelligence (AI), in which machine-learning (ML) models “learn” how to make decisions based on patterns found in large sets of patient data. This has in turn helped improve the accuracy of medical diagnoses, as well as accelerate the research and development of much-needed medicines.
However, experts in recent years realized that the traditional process of developing machine learning applications through the centralized collection of data is insufficient, as effective ML models for healthcare require more data than what would be freely shared due to issues in security and privacy. These challenges have prevented AI from taking the healthcare industry to the next level, where models that achieve clinical-grade accuracy can only be derived from sufficiently large, diverse, and curated datasets.
To democratize AI and reap the benefits of data in healthcare, there is a need for a training method for ML models that is not subject to the risks of sharing sensitive data outside of the institution that holds it. Federated learning provides such a method.
Centralized learning is no longer sustainable in healthcare
Centralized learning has long been the traditional norm in AI modelling. This method involves collecting datasets from various locations and devices, then sending it to a centralized location where the ML model training occurs.
This leads to several risks. Firstly, data stored at a single location can be stolen and exposed, causing huge liabilities to the institution responsible for storing it. Secondly, data owners might not even want to share their raw data in the first place. Though the data owners may be willing to have it used for training, the raw data itself may be too sensitive to share.
Security and privacy concerns also make it difficult to scale globally, especially with questions on data ownership, intellectual property (IP), and compliance with the varying regulations from countries around the world.
The concerns outlined above lead to fewer institutions contributing data. This in turn hinders the machine learning model from learning from a diverse and augmented set of data obtained from different institutions and geographical locations, which leads to inaccurate and biased data insights.
What federated learning brings to the table
The main idea behind federated learning is to train a machine learning model on user data without the need to transfer that data to a single location. This involves moving the training computations to the infrastructure at the data-owning institution, instead of moving the data to a single location for training. A central aggregation server is then responsible for aggregating the insights that result from the training computations of multiple data owners.
Federated learning has training iterations performed on local devices, ensuring that Vietnamese data remains stored here in Vietnam, bringing the benefit of not compromising or exposing the original data when data is in flight. This means that data remains with the owner, while still being utilized to create global insights. Local model parameters resulting from data owner training are sent to a central server, which aggregates them to form the next global model, and later shared to all participants.
Already, federated learning has made a difference by using state-of-the-art AI to better detect brain tumors. Since 2020, Intel and the University of Pennsylvania have conducted the medical industry’s largest federated learning study. With datasets from 71 institutions across six continents, the study demonstrated the ability to improve brain tumor detection by 33 percent.
Building a robust foundation for federated learning starts with trust
With so much riding on data, it is imperative that Vietnamese organizations have a robust data security strategy in place. Key to this is to keep sensitive data in the cloud inside an access-restricted enclave, commonly known as a Trusted Execution Environment (TEE). Privacy protections like these are critical to providing continuous protection of workloads with regulatory requirements or other sensitive data in distributed networks.
As computing moves to span multiple environments – from on-premises to public cloud to edge, organizations need protection controls that help safeguard sensitive IP and workload data wherever the data resides, as well as to ensure that remote workloads are executing with the intended code. This is where confidential computing comes in. Unlike traditional encryption for data at rest or in transit, confidential computing relies on a TEE for enhanced protection and privacy of the code to be executed and the data in use.
Confidential computing means datasets can be processed much more securely, and the risk of attacks can be reduced by isolating code and data from outside incursions. As the most researched and deployed confidential computing technology in the data center today, Intel® Software Guard Extensions (Intel® SGX) offers a hardware-based security solution that helps protect data in use via a unique application-isolation technology.
With a hardware-based security foundation, previously vulnerable attack surfaces can be strengthened to not only protect against software attacks, but also help eliminate threats against data in-use. Organizations can therefore have a peace of mind that their machine learning model can safely use different datasets, and train algorithms with them while remaining compliant with regulations and security.
Future of federated learning
By enabling ML models to gain knowledge from ample and diverse data that would otherwise be unavailable, federated learning has the potential to bring significant breakthroughs in healthcare, improve diagnosis, and better address health disparities.
While we are still at the beginning of exploring federated learning, it holds great promise by bringing organizations more closely together to collaborate and solve challenging problems, while mitigating issues related to data privacy and security. In fact, federated learning can stretch its application to beyond healthcare, with great possibilities in areas such as Internet of Things, fintech, and much more.
The future of federated learning will bring AI applications to the next level, and we are just scratching the surface of its true potential.